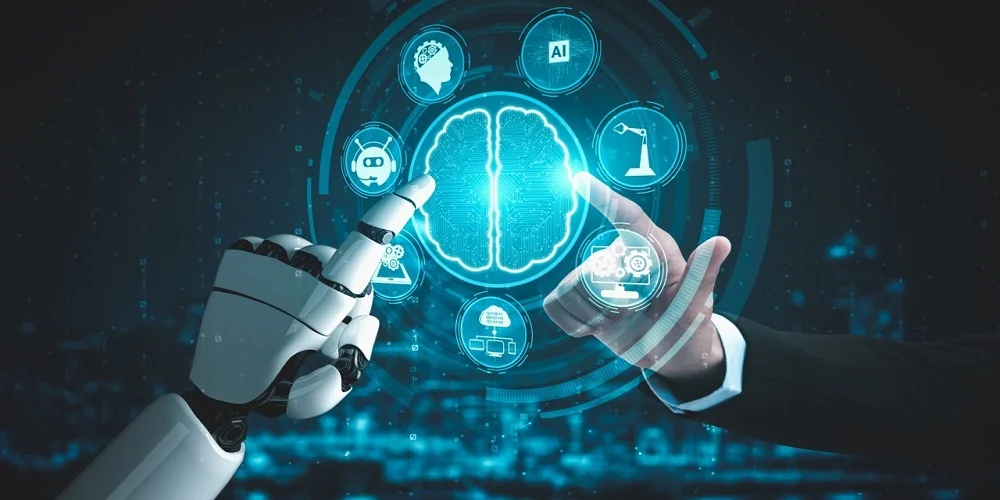
As a forward-thinking business leader, you understand that Artificial Intelligence will fundamentally transform operations and service delivery. Generative AI, in particular, is enabling companies to scale in ways never before possible. These self-learning systems can generate synthetic data, images, videos, designs, and even software code with high realism and consistency. Leaders are taking notice and starting to invest in and implement Artificial Intelligence to drive innovation and gain a competitive advantage.
This article will explore how generative AI works and share examples of companies implementing it. From enhancing product design to boosting marketing effectiveness to accelerating software engineering, AI is fast becoming essential for ambitious organisations looking to leverage technology to uncover new growth opportunities. The future is here, and generative AI may well be the most transformative technology of the next decade. Are you ready to harness its power?
Understanding Generative AI and its Capabilities
Generative AI uses machine learning algorithms to generate videos, images, text, or audio. Unlike traditional AI, which analyses existing data, generative AI creates unique, original content that can mimic human-created works.
Audio Generation
The AI learns patterns in audio data and creates new audio clips. This type of generative AI could be used to generate voiceovers, background music, or the ambient sounds of different environments. When combined with human judgment, it can augment and scale human work ethically and inclusively. Companies across industries leverage generative AI services for automated and semi-automated content creation, with applications for marketing, product development, gaming, and more.
Text Generation
The AI has been trained on massive text datasets to learn patterns in language and can generate new text in a particular style. Generative AI can generate coherent paragraphs of text, like news articles, product reviews, or even poetry.
Image Generation
Generative AI can also create photorealistic images, from human faces to landscapes to artwork. The AI has learned from huge datasets of images and can generate new, unique images in a particular style. For example, it provides product photos for e-commerce, creates character images for gaming, or generates artwork.
Real-World Examples of Companies using Generative AI
Forward-thinking companies are leveraging generative AI to enhance various business functions. Two real-world examples of companies effectively utilising generative AI are:
Anthropic
Anthropic, an AI safety startup based in San Francisco, uses Constitutional AI to generate conversational AI systems. Their techniques generate AI models that are safe, honest, and beneficial to humanity.
For example, Anthropic's AI model would refuse to send unwanted emails or generate false information, as those acts would go against its core purpose. The company's research into aligning language models and neural networks with human values has the potential to improve AI systems vastly.
DeepMind
DeepMind, a British AI company, created AlphaGo - software that plays the game Go superhumanly. AlphaGo uses deep neural networks and reinforcement learning to master the complex game.
In 2016, AlphaGo defeated Lee Sedol, the world's best Go player, in a five-game match. DeepMind's work with AlphaGo demonstrated how generative AI can be applied to complex problems and yield human-level performance.
The examples of Anthropic and DeepMind show how generative AI enables systems to perform tasks traditionally requiring human intelligence. As companies continue to integrate AI into their products and services, the future possibilities are endless. With proper safeguards and oversight, it may transform how we live and work.
Best Practices for Integrating Generative AI into your Business

Integrating generative AI into your business processes requires strategic planning and execution. To leverage successfully, consider the following best practices:
Start with a Pilot Program
Implementing a pilot program allows you to test generative AI on a small scale before an organization-wide rollout. Choose a low-risk, non-critical business process to pilot the technology. Measure key performance indicators like output, cost, speed, and quality to determine if it can scale to other areas.
Provide Adequate Training Data
Generative AI models require massive amounts of data to learn patterns and relationships. Work with internal teams to gather relevant data or purchase additional datasets to supplement your data. The more high-quality data you can provide, the more accurate outputs will become.
Leveraging AI RFP solutions can help gather and analyze vast amounts of proposal data, providing the necessary insights to train your AI models more effectively.
Continuously Monitor and Improve
Once implemented, monitor how generative AI is impacting key metrics. Look for any drops in performance or quality and make adjustments as needed. It may take several iterations of feedback before the generative AI is optimised for your specific use case.
Augment Human Workers
Generative AI should enhance human capabilities, not replace them. Use the technology to automate repetitive, mundane tasks so employees can focus on more engaging, complex work. Look for opportunities to combine AI and human judgment for the best results.
Address Ethical Concerns
Some generative AI use cases raise ethical issues around bias, privacy, and job disruption. Develop guidelines for responsible AI use, provide transparency into how models work, and consider using techniques like constitutional AI to build more trustworthy systems. With proactive risk management, you can deploy generative AI to benefit your business and customers.
Conclusion
By implementing generative AI that can generate images, videos, text, and more, businesses can scale their creative efforts, gain valuable big data insights, and improve experiences. While AI is still a developing field, its potential to enhance human creativity and shape the future of work is undeniable. Companies that fail to explore how they can benefit from generative AI risk being left behind.